
Red Teaming Challenges
About Our Red Teaming Challenges
What is Red Teaming?
Red teaming is a critical approach to assessing and improving the safety and effectiveness of technologies, particularly in the rapidly evolving field of AI. It involves deliberately testing a system to identify vulnerabilities, limitations, and potential areas for improvement. While often conducted in private by AI developers, this process can benefit from broader, more inclusive participation.
By involving diverse stakeholders, such as civil society groups and policymakers, red teaming fosters democratic oversight and helps shape smarter, evidence-based standards and regulations. Humane Intelligence, in collaboration with Seed AI and AI Village, exemplified this approach by hosting the first public red teaming event for closed-source AI models at DEF CON 2023, demonstrating the value of collective feedback in addressing complex challenges like bias and societal impact.
Stay tuned for upcoming red-teaming events.
Bias Bounty 2 Winners!
We are thrilled to announce the winners of our second Bias Bounty Challenge! This challenge was focused on developing computer vision models capable of detecting, extracting, and interpreting hateful image-based propaganda content often manipulated to evade detection on social media platforms.
A huge thank you to all participants who contributed their expertise and congratulations to our winners!.
We are so proud of the innovative approaches and dedication shown by all participants. Stay tuned for our upcoming Bias Bounty Challenge 3 to continue pushing the boundaries of algorithmic assessment and ethical AI development.
Advanced:
Mayowa Osibodu
TUESDAY
Devon Artis
Intermediate:
Gabriela Barrera
Blake Chambers
Chia-Yen Chen
Bias Bounty 1 Winners!
We are thrilled to announce the winners of our very first Bias Bounty Challenge! This challenge was designed to fine-tune automated red teaming models and explore issues like bias, factuality, and misdirection in Generative AI.
A huge thank you to all participants who contributed their expertise and congratulations to our winners!.
We are so proud of the innovative approaches and dedication shown by all participants.
Advanced:
Yannick Daniel Gibson (Factuality)
Elijah Appelson (Misdirection)
Gabriela Barrera (Bias)
Intermediate:
AmigoYM (Factuality)
Mayowa Osibodu (Factuality)
Simone Van Taylor (Bias)
Beginner:
Blake Chambers (Bias)
Eva (Factuality)
Lucia Kobzova (Misdirection)
Red Teaming Types
Expert Red-Teaming
Small group assessments by invited experts in non-technology fields.
Goals:
-
Testing narrow harms and point issues.
-
Supplementing internal red teaming efforts with external expertise (e.g, medical, legal).
Public Red-Teaming
At-scale challenges conducted by invitation or fully open to a wide range of individuals with unique expertise.
Goals:
-
Diffuse harms.
-
Gathering data en-masse to identify systemic issues vs point issues.
Two broad forms of AI Safety/Security testing
1. Unintended consequences: Individuals who are interacting with the AI systems are using them ‘naturally’ - no intent to break in or hack. However, an adverse event occurs.
2. Malicious attacks: The user is intentionally interacting with the system with the goal of attacking the system in some way.
Why is this important?
-
Your red teaming approach changes - you may not engage in prompt injections for testing unintended consequences
-
Harms from unintended consequences may be more diffuse/abstract (eg difficult to quantify the impact of having images of CEOs be all white men) than malicious attacks (eg, stealing credit card info
Steps for red-teaming
1. Determine your “rules of engagement”: Your rules of engagement state what you are testing against. This could be a product’s ToS, or a law, or guidelines.
2. Identify your population and testing audience (if relevant): Are you testing AI models in a specific usecase? Can you identify an audience of people to test it?
3. Establish clear challenges and require evidence/proof: Define your challenge narrow enough to provide direction, but broad enough to allow free-form testing
4. (Optional) Align output to an existing taxonomy of harms: Red-teaming works best when it is linked to some accountability framework or taxonomy of harms. This could be similar to your rules of engagement ground truth but would not be the identical document. Existing taxonomies can help with understanding severity of harm, for example.
Methods of prompt injection attacks for malicious attacks
1. Direct prompt injection attacks: A prompt injection attack aims to trick AI systems into disclosing sensitive information, or providing dangerous information, such as how to build weapons or produce drugs. These attacks can result in reputational damage, as the tool's output would be associated with the company hosting the system.
2. Indirect prompt injection attacks: AI systems can read webpages and provide summaries. Inserting prompts into a webpage, so that when the tool reaches that part of the webpage, it reads the malicious instruction and interprets it as something it needs to do, constitutes an indirect prompt injection attack.
3. Stored prompt injection attacks: When an AI model uses a separate data source to add more contextual information to a user's prompt, that source could include malicious content that the AI interprets as part of the user's prompt.
4. Prompt leaking attacks: Prompt leaking aims to trick the AI tool into revealing its internal system prompt, especially if the tool is designed for a particular purpose. Such tools' system prompts are likely to have highly specific rules, which might contain sensitive or confidential information.
Events
The Chief Digital and Artificial Intelligence Office (CDAO) + Humane Intelligence: Advancing AI Assurance in Military Medicine through Crowdsourced Red Teaming
January 2, 2025
Humane Intelligence partnered with the Department of Defense’s Chief Digital and Artificial Intelligence Office (CDAO), the Defense Health Agency (DHA), and the Program Executive Office, Defense Healthcare Management Systems (PEO DHMS) to conduct a groundbreaking red-teaming initiative. Focused on Generative AI (GenAI) applications in military medicine, the effort explored vulnerabilities and biases in clinical note summarization and medical advisory chatbot systems. Over 200 participants, including clinical providers and healthcare analysts, tested three popular Large Language Models (LLMs), uncovering more than 800 critical findings. These insights are helping to establish benchmark datasets and shape DoD policies for the responsible adoption of GenAI in military healthcare, ensuring safety, equity, and improved care for service members. This effort exemplifies the power of crowdsourced testing in fostering resilient, ethical, and effective AI technologies.
AISA Australian Cyber Conference 2024
November 26, 2024
Humane Intelligence, in partnership with AISA and CSIRO, hosted the AI Village at the 2024 AISA Australian Cyber Conference in Melbourne, Nov 26-28. The event, Australia’s largest on AI security, featured interactive sessions like a red-teaming exercise led by Fellow Fariza Rashid and a capture-the-flag challenge targeting vulnerabilities in Large Language Models (LLMs). Inspired by the 2023 White House AI Red Teaming, it explored bias, hallucinations, and prompt injection in AI systems.
UNESCO - technology facilitated gender based violence
November 25, 2024
Humane Intelligence partnered with UNESCO in Paris for a red-teaming exercise on Technology-Facilitated Gender-Based Violence (TFGBV). Held on the International Day for the Elimination of Violence Against Women and Girls, senior diplomats and UNESCO experts tested generative AI models to identify vulnerabilities, biases, and safety gaps. This collaboration builds on UNESCO’s extensive work on gender and AI, advancing practical solutions for fair, inclusive AI systems.
Singapore IMDA red teaming on multilingual and multicultural biases
December 9, 2024
Humane Intelligence, in collaboration with AI Singapore and global leaders like Anthropic, AWS, Cohere, Google, and Meta, launched the first regional AI Safety Red Teaming Challenge in Asia. Participants from nine countries, including sociologists, linguists, and cultural experts, evaluated four LLMs to detect bias and stereotypes in English and regional languages. This groundbreaking initiative addresses gaps in AI safety evaluations, which often focus on Western contexts, and seeks to develop a common methodology for safer, culturally sensitive AI models. An evaluation report summarizing findings will be published in early 2025.
FEMYSO - AI and Islamophobia
September 21, 2024
Humane Intelligence and FEMYSO joined forces at the European Action Day Against Islamophobia Conference 2024 to address AI ’s role in perpetuating anti-Muslim bias. Through discussions and an Islamophobia Red Teaming Workshop, participants examined political shifts impacting Muslim communities and tested Gemini, ChatGPT, and Claude for harmful stereotypes.
NIST red teaming exercise
September 9, 2024
Humane Intelligence, supported by the U.S. National Institute of Standards and Technology (NIST), conducted a nationwide AI red-teaming exercise to test and evaluate generative AI systems' robustness, security, and ethical implications. Open to U.S.-based individuals and model developers, the initiative advanced AI resilience and trustworthiness through rigorous adversarial testing and analysis.
Red Teaming Exercise With Journalists
September 9, 2024
Humane Intelligence and Compiler hosted an AI reporting workshop at Northwestern University’s Medill School, training 10 journalists on HI’s no-code evaluation platform to investigate generative AI’s societal impacts. Participants explored AI regulation, data journalism, and machine learning, producing original stories for a special edition of Compiler.
Autodesk University Red Teaming Exercise
November 13, 2023
At Autodesk University 2023, Humane Intelligence partnered with Autodesk to conduct a two-day red-teaming exercise with architects and engineers. Participants tested generative AI in design applications, assessing structural integrity, creative capability, and potential IP violations of AI-generated outputs, such as modern art museum designs and electric vehicles. Insights aim to ensure responsible, ethical AI use in creative industries.
Royal Society Red Teaming Exercise
October 25, 2023
Humane Intelligence, in partnership with the Royal Society, hosted a red-teaming event where invited COVID-19 and climate scientists tested Meta's Llama 3.0 for mis- and disinformation vulnerabilities. Participants, adopting the personas of misinformation actors, explored how GenAI could amplify these challenges. Findings were shared with Meta to improve the safety of scientific LLMs.
DEFCON Red Teaming Exercise
August 14, 2023
At DEFCON 2023, Humane Intelligence partnered with Seed AI and DEFCON AI Village to host the largest-ever Generative AI public red teaming event. Over 2.5 days, 2,244 participants evaluated 8 large language models (LLMs) through 17,000+ conversations on topics like cybersecurity, misinformation, and human rights. This groundbreaking event demonstrated how public red teaming can provide nuanced insights into AI biases and social harms, complementing traditional corporate evaluations. Partners such as Meta, Google, and OpenAI contributed, along with policy groups like the White House OSTP and NIST.Findings and the anonymized dataset are available on our GitHub, advancing collaboration on AI safety and accountability.

Stay in touch!
Sign up to stay up to date on upcoming challenges, events and to receive our newsletter.
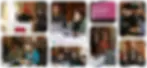
FAQs
Bias bounties are not only a vital component of assessing AI models but are a hands-on way for people to critically engage with the potential negative impacts of AI. Bias bounties are similar to the more well known bug bounties within the cybersecurity community. But instead of finding vulnerabilities in the code, bias bounties seek to find socio-technical harms. These harms can include factuality issues, bias, misdirection, extremist content, and more. Bias bounties are narrowly scoped challenges, focused on a particular data set and problem.
Bias bounties complement red teaming exercises, which are broader in scope and primarily focused on breaking the safety guardrails of the AI model.
Each of our challenges will have a set start and end date, with the majority of challenges running for at least one month. Most often the datasets will be hosted on Humane Intelligence’s GitHub, unless the data contains sensitive information. All of our challenges involve cash prizes and how the prizes will be distributed amongst winners in different skill levels will be shared in the challenge overview.
We are currently exploring future bounty challenges in these areas: hiring, healthcare, recommender systems, insurance, policing, policymaking, gendered disinformation, elections, disparate impacts, disability, counter-terrorism, and islamophobia.
Themes are selected with a variety of factors in mind, such as impacts on real world issues, access to data, needs of our partner organizations
If your organization, agency, or company is interested in having your AI models assessed, please contact us. We can coordinate around building a public red teaming challenge, a bias bounty focused on a specific use case, or a private assessment done internally.
If your organization, agency, or company is interested in having your AI models assessed, please contact us. We can coordinate around building a public red teaming challenge, a bias bounty focused on a specific use case, or a private assessment done internally.
Our bias bounty challenges run the gamut from the more technical that involve coding to the more accessible that involve generating prompts. Our technical challenges often include various skill level options so a wider range of people can join. Most often our challenges do not require any pre-requisite knowledge to participate.
Some of our challenges can be done as a team but this is not required. You are responsible for organizing your team, dividing the work amongst yourselves, and, if applicable, dividing any winnings amongst yourselves. We have dedicated channels on our Discord server for each challenge and for finding a partner.
The scope of each challenge will be unique, so be sure to read through the specifics of each challenge to assess what skills are needed for each challenge level to determine your abilities.
We are eagerly seeking outreach opportunities with organizations, universities, and academic institutions around the world to ensure that we have a diverse range of participants. If you’d like to put us in touch with such a group, send us an email at hi@humane-intelligence.org
We understand that bias bounties are a new concept to many people, so we are actively creating a repository of resources for people to learn.
Discord Community
On our Discord server, there will be channels created for each of our bias bounty challenges for participants to ask questions. Additionally, there is a research channel where community members share the latest in red teaming and bias bounty tactics.
Landscape Analysis
We have an ever-evolving database featuring a landscape analysis of AI evaluations, which includes various organizations (academic, NGO, business, network, government, and others) and resources (papers, tools, events, reports, repositories, indexes, and databases). Users can also search for different AI evaluation categories, such as Benchmarking, Red-Teaming, Bias Bounty, Model Testing, and Field Testing.Tutorial Videos
For our first bias bounty challenge, one of our Humane Intelligence Fellows created a tutorial video series that walks complete beginners through the process of downloading datasets, creating a coding notebook, analyzing the data, and submitting challenge solutions. While the specifics of challenges will change, the general processes outlined in these videos will remain the same.
Challenge Submission Guidelines
Each of our bias bounty challenges will include an overview, suggestions on how to tackle the issue, and the criteria that will be used for grading. Most often your submission data will be incorporated into a coding notebook that contains code written by our data scientists to assist with the grading.
We understand that bias bounties are a new concept to many people, so we are actively creating a repository of resources for people to learn.
Discord Community
On our Discord server, there will be channels created for each of our bias bounty challenges for participants to ask questions. Additionally, there is a research channel where community members share the latest in red teaming and bias bounty tactics.
Landscape Analysis
We have an ever-evolving database featuring a landscape analysis of AI evaluations, which includes various organizations (academic, NGO, business, network, government, and others) and resources (papers, tools, events, reports, repositories, indexes, and databases). Users can also search for different AI evaluation categories, such as Benchmarking, Red-Teaming, Bias Bounty, Model Testing, and Field Testing.Tutorial Videos
For our first bias bounty challenge, one of our Humane Intelligence Fellows created a tutorial video series that walks complete beginners through the process of downloading datasets, creating a coding notebook, analyzing the data, and submitting challenge solutions. While the specifics of challenges will change, the general processes outlined in these videos will remain the same.
Challenge Submission Guidelines
Each of our bias bounty challenges will include an overview, suggestions on how to tackle the issue, and the criteria that will be used for grading. Most often your submission data will be incorporated into a coding notebook that contains code written by our data scientists to assist with the grading.
Each bias bounty will have a specific grading criteria that will be released at the launch of the challenge, in addition to submission instructions. The grading criteria will often be different for each skill level. Submissions will be graded by the Humane Intelligence staff following this criteria.
To see examples of our previous grading criteria: Bias Bounty 1 and Bias Bounty 2 (Intermediate and Advanced).
We aim to grow the community of practice of AI auditors and assessors; one way we strive to do so is through sharing what participants learned by completing challenges , as well as the broader insights learned about the particular issue area of the challenge. Participants are also encouraged to share their insights in our Discord community.
Additionally each of our challenges will include details about how these learnings will be used by us and our external partners to make AI more equitable and safe.
The grading and submission instructions for challenge 1 are here
You can only submit to one competition, so choose wisely.
No.
Yes.
Yes. Feel free to post on our discord channel to look for a partner. However, if you win, only the submitting account/person accepts the prize and you will be responsible for dividing amongst yourselves.
You can post to our discord channel to look for a partner.
Other Questions?
Find us on our Discord channel